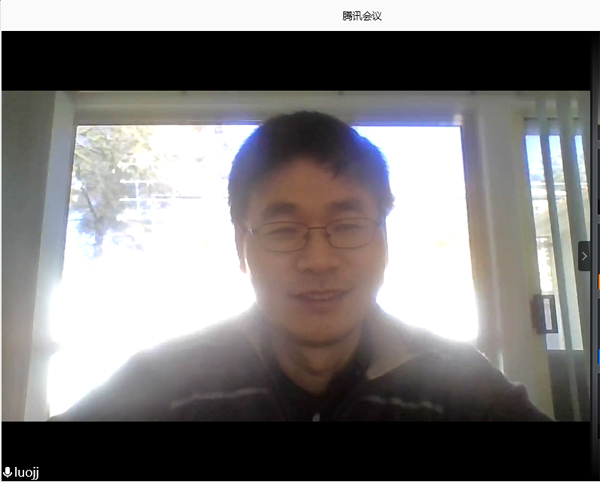
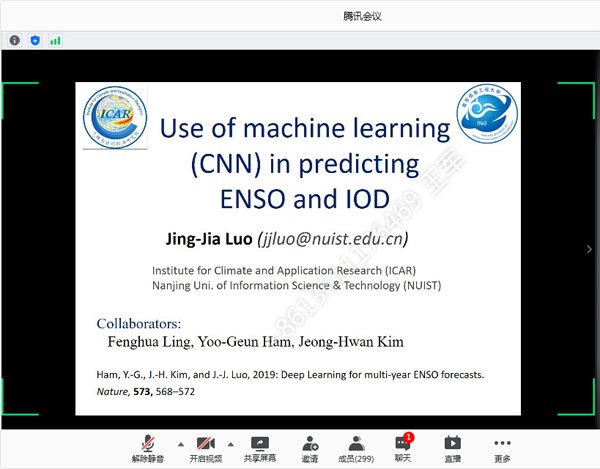
Use of machine learning technique in predicting ENSO and IOD
罗京佳 教授
南京信息工程大学
邀请人:段晚锁 研究员
报告会主持人:段晚锁 研究员
2020年6月19日(星期五)10:00
腾讯视频会议 (会议ID: 219 815 381)
会议链接: https://meeting.tencent.com/s/lEaohbS6UEy5
会议直播: https://meeting.tencent.com/l/Cwwdk0inwpZO(不限人数)
报告摘要:
Skillful forecast of ENSO and IOD events at lead times beyond one year remains a tough challenge. Here we show that a statistical forecast model employing a deep-learning approach produces skillful ENSO forecasts for up to one and a half years in advance. To circumvent the limited amount of observational data, we first train a convolutional neural network (CNN) model by use of historical CMIP5 simulations, which is further tuned based on observational reanalysis during 1871-1973. The CNN skill in predicting ENSO and its diversity during 1984-2017 outperforms the current dynamical forecast systems including SINTEX-F. In addition, the CNN model displays good skill in predicting the IOD indices, competing with dynamical models. A heat map analysis indicates that the ocean precursors selected by the CNN model for the long-lead prediction of ENSO and IOD events are consistent with existing physical understandings. Despite many caveats, the machine learning technique shows a big potential in helping improve our understanding and prediction of tropical climate.
报告人简介:
罗京佳,南京信息工程大学教授、ICAR院长,CLIVAR太平洋区域委员会委员。长期从事气候机理,模式研发,预测和应用等方面的研究,取得了多项国际先进成果,2008年以来发布提前2年的厄尔尼诺实时预测信息,具有广泛的国际影响力。在太平洋年代际气候变化机理,热带海盆间的相互作用等方面有多项原创性成果,共发表Nature、Science、PNAS等论文110余篇,Google?Scholar统计的文章总引用数超过9600次。荣获多项国内外奖励,包括日本科技文化体育部青年科学家奖等。